MEA-MIA
Innovative solutions to improved the future commute experience in San Diego.
Overview
Timeframe: 10 Weeks
Tools: Adobe Illustrator, Invision, Figma, Surveys
Role: UX Researcher, Product Designer
Background & Problem Space
In partnership with organizations such as UC San Diego Design Lab and Design Forward San Diego, D4SD (Design for San Diego) was a 10 week long design competition focused on innovative solutions for the future roads of San Diego. Participants were encouraged to find a solution in one of the following 4 paths.
-
Pedestrian Safety
-
Accessibility
-
Commuter Experience
-
Autonomous Vehicle
Field Observations & Interviews
We were first inspired by the city of San Diego's Vision Zero initiative which aims to reduce the number of commuter casualties to 0. We began field observations at some of the "fatal 15" Intersections that are known for the highest number of pedestrian injuries and deaths in the past year.
We noticed a general pattern of drivers rushing into cross walk for right turns while failing to check for pedestrians or cyclist. This resulted in many abrupt stops and it became clear why those intersections could be so deadly if the vehicles were going a little faster or if the vehicles behind are following too closely. What was interesting on the flip side was despite being physically vulnerable, pedestrians were still jaywalking, causing safety concerns and disrupting traffic as well.
But why would pedestrians do this? We conducted interviews at the intersections and found that pedestrians often did not think the crosswalk was safer than the street because drivers do not pay attention anyways.
We also conducted interviews in the streets of downtown La Jolla with lots of pedestrians, hectic traffic and a lack of traffic signals. Most people reported having to pay extra attention. For example pedestrians reported drivers being on their phones and being distracted while drivers reported pedestrians disregarding vehicles on the road assuming they will always be given the right of way.
An interviewee bought up a driving behavior tracking service by Progressive, where one’s driving patterns affected their insurance rates. She claimed that if more drivers adopted this, they would drive more carefully. Another interviewee however was against this concept because she thought that driving was “too reactionary and situational.” We realized that speeding, braking hard, and quick lane changes don't equate to bad driving since they are sometimes necessary to avoid accidents.
Surveys
We also needed quantitative data from surveys. The results indicated that people knew distractions like cell phones and conversations were the main causes for accidents, but they continue to engage in distracted driving.
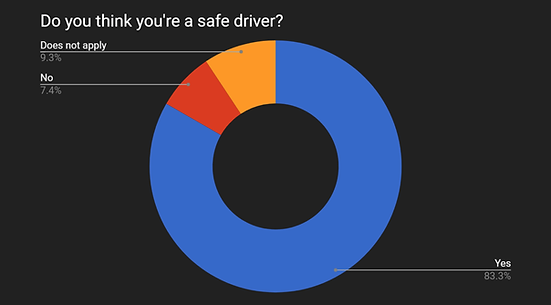
Most people also thought they were good drivers despite most of them also self reporting unsafe driving behaviors and habits. This is similar to our interview findings where both drivers and pedestrians reported the other group as being at fault while still engaging in dangerous traffic maneuvers themselves.
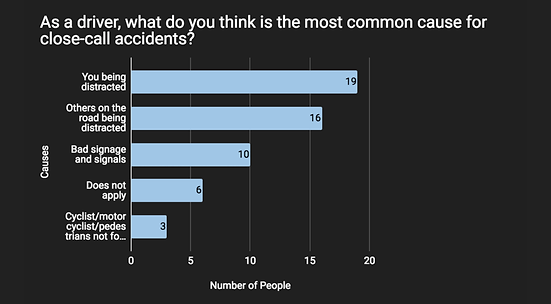
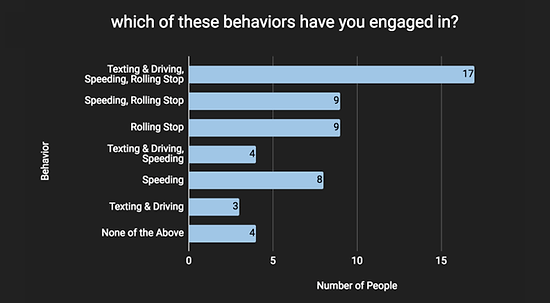
Our insight was that people's confidence in their own driving skills and need to prioritize their own agenda before the safety of others were the root causes of accidents. I also realized that being in a physical property that I owned (the car) gave me a sense of privacy and protection that de-personified others.
Ideating
We began ideating on ways to increase empathy between drivers and pedestrians on the road. But changing behavior is difficult.
Empathy is so intrinsic and subtle that it varies from person to person. That's why strict traffic rules and laws exist, because it is impossible to make everyone empathize at the same level. This made it difficult to generalize any solution to a broad user base. We needed to take a different angle towards improving safety on the road.
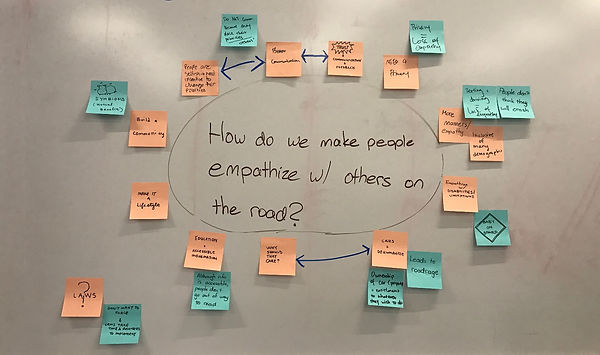
A co-instructor gave us insights on her research on AVs. She found that driving become more passive over time because drivers get accustomed to technology and the environment. They slowly develop motor functions that react almost subconsciously to environmental cues. This can be linked to the survey results where a large number of users had high confidence in their driving abilities. Familiarity = confidence. Thus, we had to figure out how to break drivers out of this passive state of driving, possibly by incorporating ways to alert them of their surroundings.
As we diverged deeper into the problem of passive/distracted driving, we noticed that many of the causes were due the driver’s dependency on vision. We converged on this point and began ideating for solutions that incorporated other senses to reduce the cognitive load off of vision. This stage was tough because many of our ideas were already integrated into vehicles that are semi-autonomous. There was also the concern of over-stimulation or even increased distractions.
We came across a DMV report on all AV related accidents; more than 90% of which were caused by humans. The AVs make the correct decisions, but in such an irregular fashion that human drivers are unable to react in time.
As we progress towards future roads driven by AV’s, there will be a long transitional period where both AVs and human drivers have to co-exist. We realized this is a bigger issue that required us to pivot to focus on AVs. AVs will always react quicker than human drivers but are less adaptive/flexible. Human errors on the road could be dealt with safely if AVs were supplied with sufficient data about how humans react to unexpected situations. Is there a way to get drivers to share their driving data to AV companies?
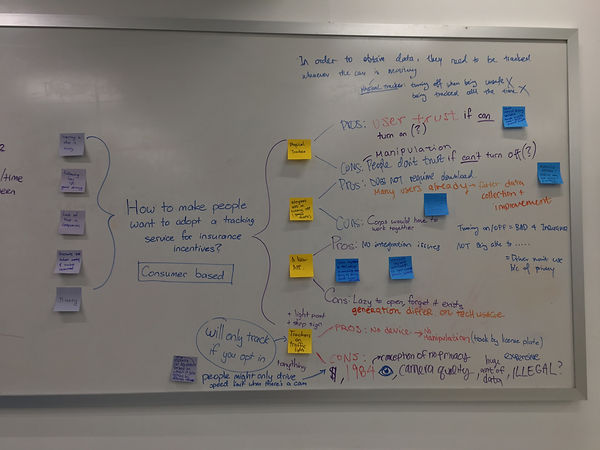
New problem statement: A Challenge that AVs are facing is how to coexist with human drivers on the road. Companies need data on human driving patterns to create a safe transition from human drivers to AVs.
Our idea at this point revolved around a service that connects AV companies to users’ driving behaviors. AV companies will pay drivers in exchange for this data depending on how much time driven. We thought it was a fair incentive that created a mutually beneficial relationship.
We also learned from industry experts and guests that companies like Uber and Tesla have open source data collected from their products so they are unlikely to pay for it.
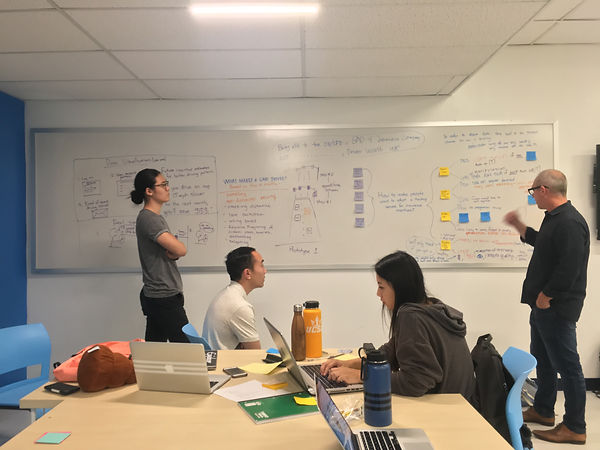
We decided to make the data open sourced and pitched the solution as a nonprofit organization, where driver incentives are paid by donations. We invited Scott Robinson as a guest to give us feedback.
He liked quality of the data because the current data available to AV companies is skewed, coming from “super-users” such as Uber drivers and Tesla owners, who do not represent the average driver. Longitudinal data from everyday users over time can reveal interesting fluctuations in driving patterns and trends.
Our Primary Stakeholders are AV companies and academic institutions who will get access to organic data from a variety of locations, letting them develop more advanced AVs for safer roads. While our Secondary Stakeholders are everyday drivers who can generate extra earnings and help improve AVs by simply drivings.
We interviewed more young drivers and most reported they would use the app and the preferred minimum amount of incentive averaged at 58¢ per hour of driving data.
Of all the professors, guests and researchers we interviewed, only a handful said they were willing to use the service. When asked why not they said the incentive isn’t significant for them to risk their privacy. However they suggested the option to donate to charities to add an intrinsic "feel good" dimension to the service for people that are not interested in the money. Regardless, this is still significant because research shows that drivers between ages 20 and 24 cause the most accidents on the road (McClellan Law).
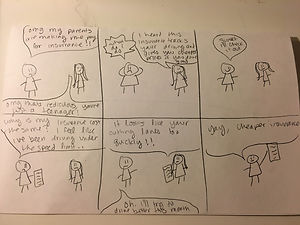
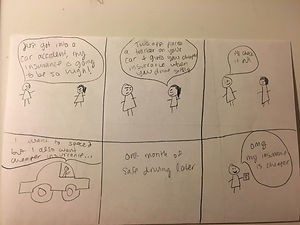
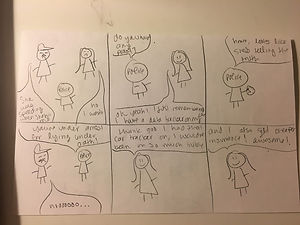
Competitors & Key Functionalities
Our competitors were companies like Progressive, Dash, Automatic, and Zubie, who have devices that pull information from the OBD-II port of cars. Their participants must purchase an additional, physical plug-in device, which the users regarded as one of the biggest breakdowns.
How did we want to address this? We thought about installing cameras in intervals on the road. This will generate significantly more data but also required too much investment in the installation of the infrastructure. So we turned to smartphones.
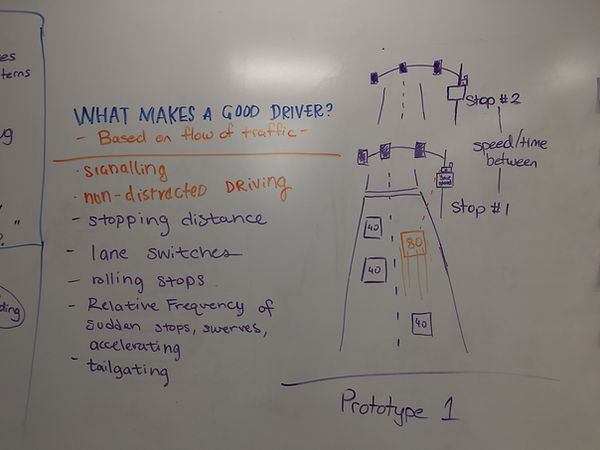
But how is MEA different? The key breakdown that we addressed better than our competitors was privacy.There were overwhelming concerns from users of our competitors that their locations were being tracked at all times. MEA does not require a physical plug in and could be disabled at the users’ will because it relies on the smartphone.
The novelty in MEA lies in targeting longitudinal data collected from everyday drivers. The app enables a visualization of individual driving trends over time, giving the dataset high ecological validity.
GPS technology on smartphones can track speed, acceleration, and braking patterns based on location services. As for micro-behaviors like lane changes and merges,the phone’s accelerometer and gyroscope are able to detect sensitive rates of change on a 3-dimensional plane by measuring linear acceleration of movement and angular rotational velocity.
Branding & Protoype

To bring even more comfort to the act of collecting sensitive data , we wanted to add a sense of ownership to the data. We did this through different ways of data visualization similar to a fitness tracker. Users can also see which institutions are using their data and what projects they have contributed to.

We followed similar design principles that finance apps followed. Apps like Venmo, SweatCoin, or bank apps all prioritized the visualization of a monetary amount on the main pages.
MEA stands for Mine, Earn, Automate.
The digital prototype was very similar to the paper prototype, aside from the donate to charity feature. Check out the interactive prototype linked here: https://invis.io/FRE6X5FEG
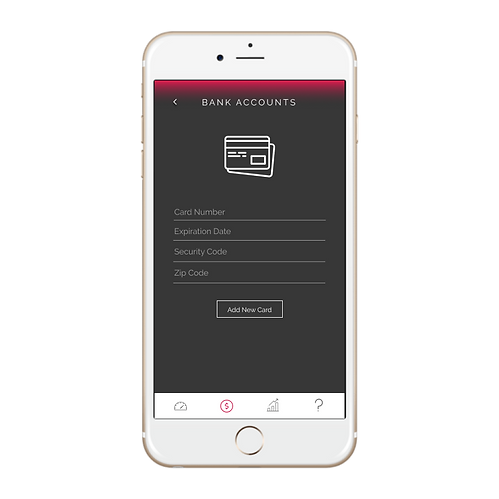
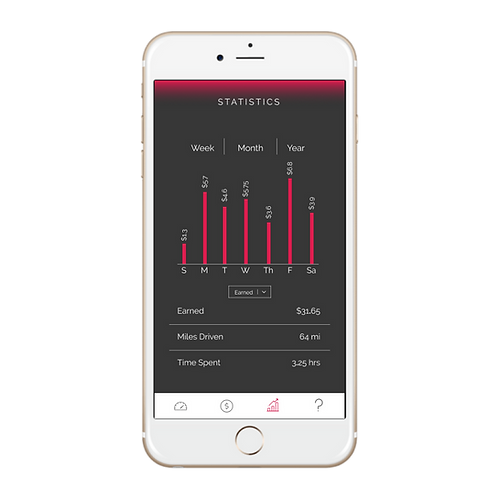
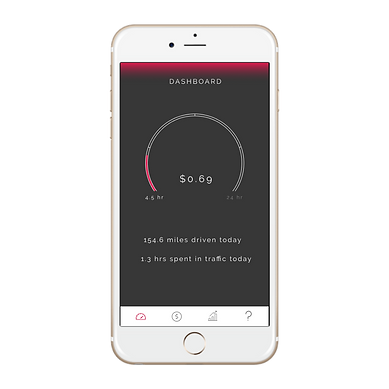
Click HERE to open the poster we created for the competition.
Click HERE to watch our promotional video of the product.
Reflection & Pivot
After the competition, we began analyzing the main critiques from the judges and itt seemed that our solution was too far-fetched and indirect. Our team highlighted a problem that would occur with the introduction of AVs. However, we were unable to provide a direct solution that has immediate impact. Our problem statement remained: “How can we better facilitate communication between human drivers and AVs.” But we needed to pivot.
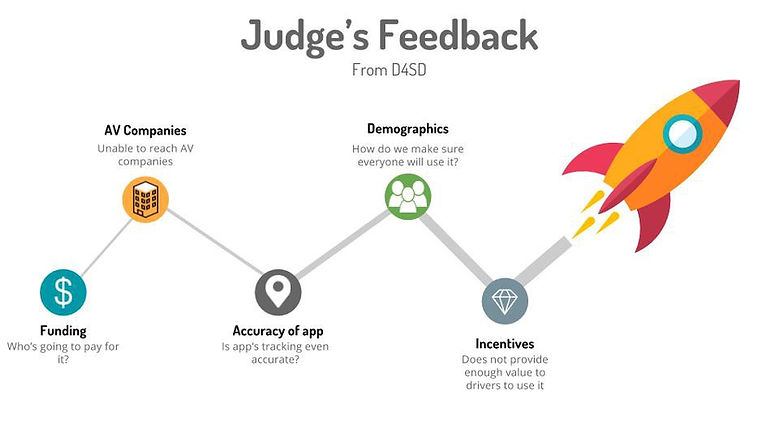
Going back to the needfinding phase, we found that California’s DMV had recorded all AV related accidents within the past 3 years. We found that a majority of the accidents were caused by either rear end or left-turns/lane changes.
More articles and data further validated our previous findings that distracted driving was still the biggest cause of accidents. But both novel and experienced drivers engaged in distracted driving so why do they cause more accidents for teens/novice drivers? In a study done by Geoffrey Underwood (2014), after conducting and eye tracking experiment on both novel and experienced drivers, he discovered that new drivers simply have not developed the necessary mental model to drive distracted.
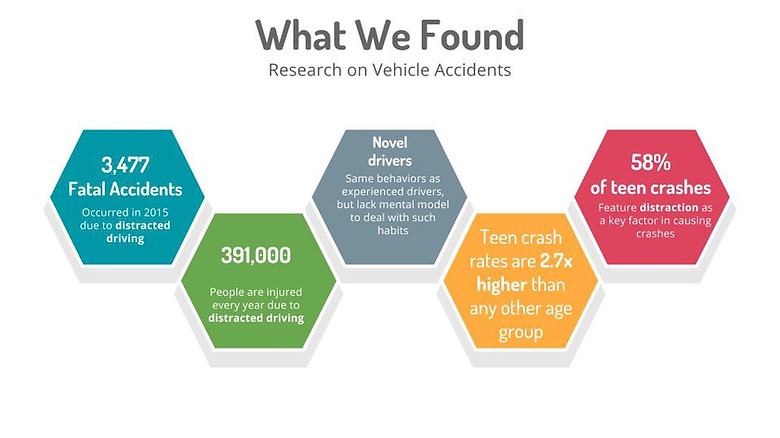
We were able to assign the causes of most accidents into 3 main reasons: Distracted driving, cognitive overload, lack of experience. With this in mind, we decided on a new problem statement:
“How can we dynamically encourage active driving depending on a specific driver's habits?”
Ideation Phase 2
With a new problem statement in mind, we began generating new ideas focused on utilizing different modalities and senses to encourage active driving.
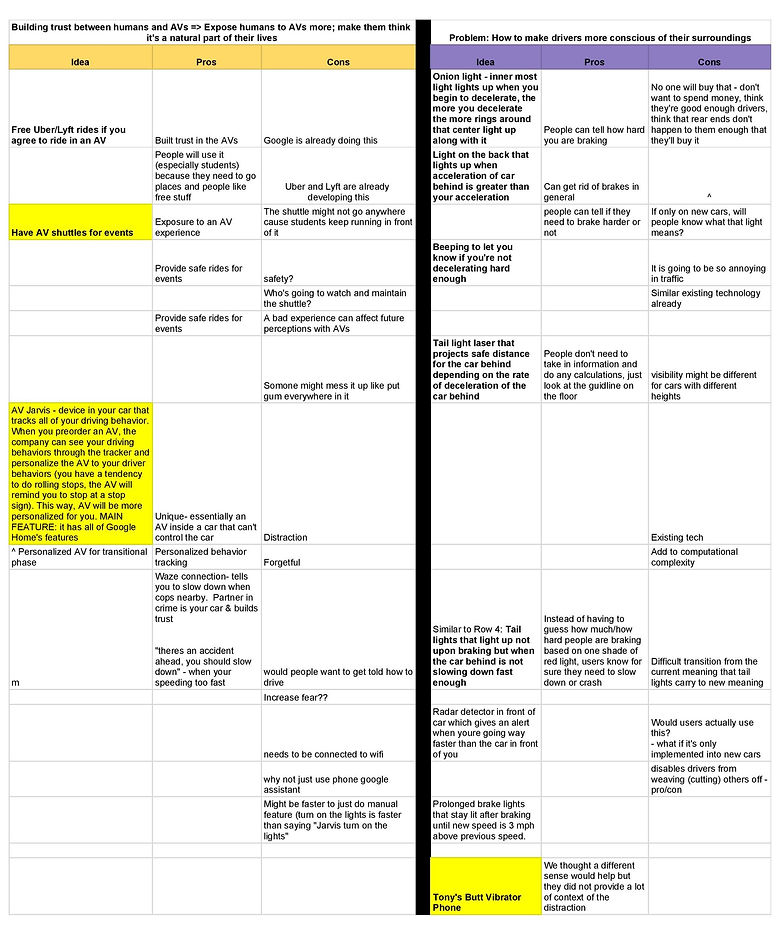
Prototyping & User Testing
We liked the solutions that relied on visual and tactile cues as those are two of the most commonly used senses in daily life. One such solution was The Onion Light.
Despite cars on the street decelerating at different rates depending on the different pressure applied on the brake pedal, the conventional tail lights only communicated one piece of information: the car is slowing down. To communicate more useful information, we imagined cars with rear sensors to capture the acceleration and deceleration rate of the car directly behind. The system will take information from both the driver's car and the car behind to decide and display the appropriate intensity of braking to the driver behind. The prototype was a graduated brake light to communicate different appropriate braking intensities.
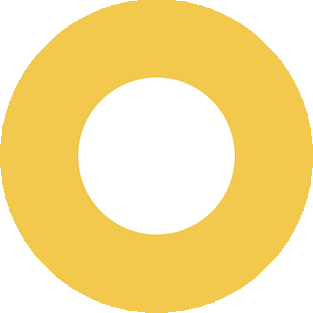
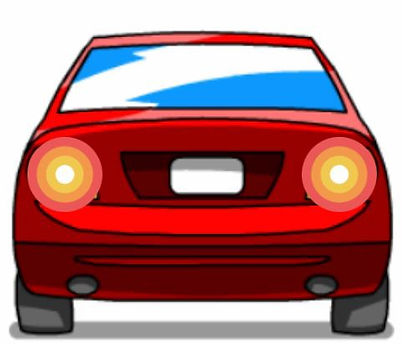
We wanted to test if drivers will understand the information the lights communicate. Upon showing them the animation, to our surprise most of them interpreted it differently. Because of the colors we used and the location of the lights, people associated it with signaling and even emergency lights. Around the same time, we found out that graduated brake lights and the act of modifying brake lights are actually illegal in most of North America.
The second solution was the Vibrating Seat. Drivers have to interact with so many dynamic elements on the road but they can only look forward. Utilizing tactile cues, we imaged cars with sensors that communicate information on the surroundings by vibrating different parts of the driver’s seat.
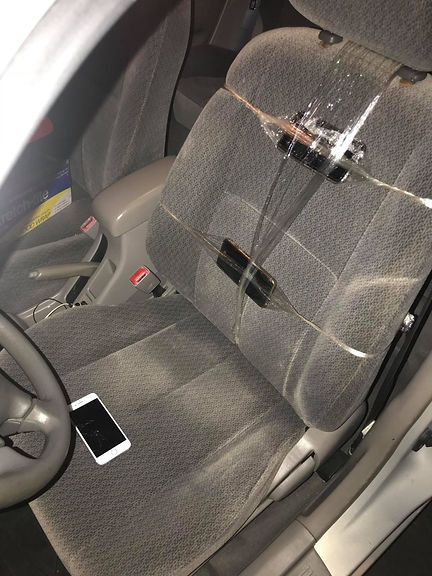
We wanted to know if people can actual distinguish among the different vibrations and remember what they mean and carry out appropriate actions in response. However users agreed across the board that it was too difficult to distinguish among different parts of the back. We discovered the theory of 2 point discrimination, stating that less sensitive areas of the body will have a harder time distinguishing between 2 different sites of stimulation.
MIA & Existing Solutions
After honing in on human’s visual and tactile senses, we decided to see if we could use audio as a way to help drivers. Inspired by Iron Man’s Jarvis, we came up with an auditory prototype that not only alerts drivers to potential obstacles, but acts as a teaching assistant to new drivers to enforce good driving habits.
So why was this idea better than a visual assistant? Since driving already spikes cognitive load, especially for new drivers, we hypothesized that providing another visual focus for the drivers would only distract them even more.
Furthermore, we discovered that there were two ways of listening: passive and active. As shown below, passive listening which we will utilize barely affects reaction time. This meant that audio cues have the potential to provide more contextual information about the road while minimizing distractions.

We decided to take MEA into a new direction: MIA (My Instructional Assistant). Instead of promoting data mining for AV companies, we decided to focus on technology that will have direct and immediate impact. We decided to utilize car sensor technology including ultrasonic, radar, lidar, and eye-tracking technology.
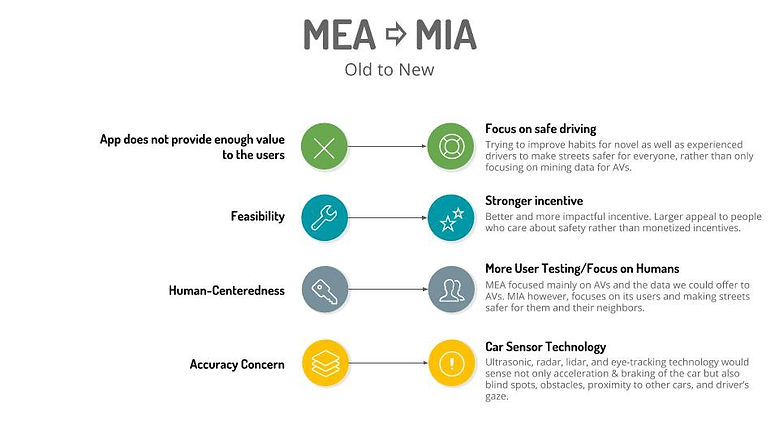
But what about our competitors?
First, we discovered Microsoft’s Cortana: Cortana is a digital assistant that was to be implemented into BMW and Nissan. Next, we looked at existing products that were designed for new drivers: LifeSaver, AT&T Drive Mode, Mojo, and Everdrive.
How is MIA different? First, these apps do not focus on teaching the driver good driving habits, instead the apps just take away the driver’s ability to use the phone. Secondly, these apps do not respect the privacy of the new drivers. The app can be set up to notify users' parents if they have a passenger, speed, or try to uninstall.
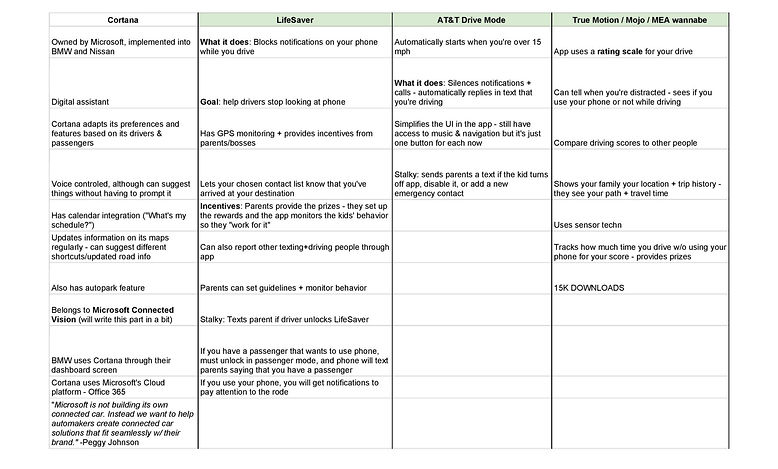
The next app, EverDrive, was similar to our original MEA concept which means it does not focus on enforcing good driving habits. The final program on our competitor list was Fleetmatics — a commercial based tracker device that is installed into trucks. However, the dashboard does not provide any real time feedback to the driver.
After conducting the research, we felt confident in moving forward with our new solution: MIA. In order to accurately assess the public’s opinion on MIA, we conducted some usability testing of the concept. The protocol we decided to adopt for the testing sessions consisted of the following:
-
Start off by sitting in the backseat
-
Emulate the audio assistant and limit distractions
-
Play notifications from MIA soundboard (spoken)
-
Relevant phrases were "played" whenever driver behavior triggered a warning/notification
-
Opt-in/Opt-out option for certain notifications
Features & User Testing
We then recorded a MIA soundboard, consisting of real time alerts and safety reminders. Below are a couple of examples.
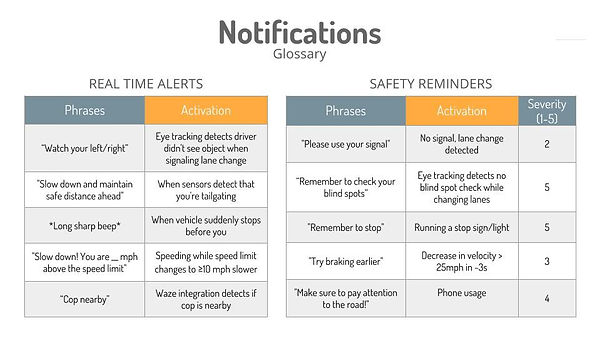
We received positive feedback from most users like wanting a way to visualize the data and keep track of their driving behaviors to actually see the improvement. They also suggested new features that we were able to make possible with sensors and eye tracking.
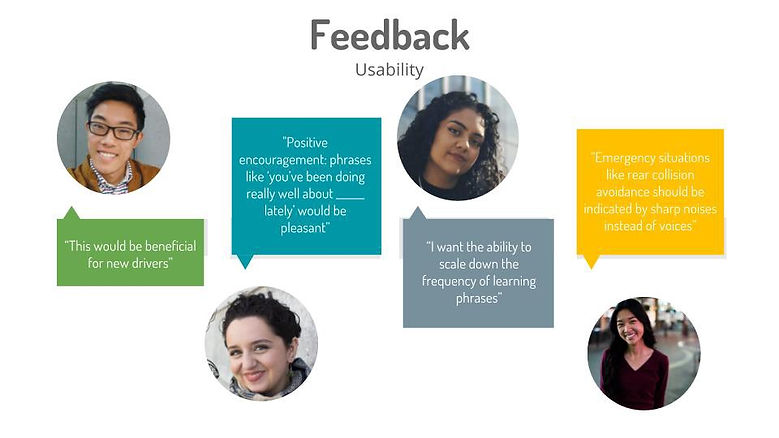
In order to increase customizability, we decided to implement an AI system that uses driving behaviors to adapt an appropriate feedback system that is unique to every user. Users wanted the options to turn on/off real time alerts and change the frequency of safety reminders. We also agree that there should be a way to minimize alerts when there is improvement in driving. However, if users had the option to completely turn off alerts, the goal to correct bad driving habits would be rendered pointless. Therefore, we opted for a machine learning algorithm that scales frequency up and down, proportional to severity & frequency of violations.
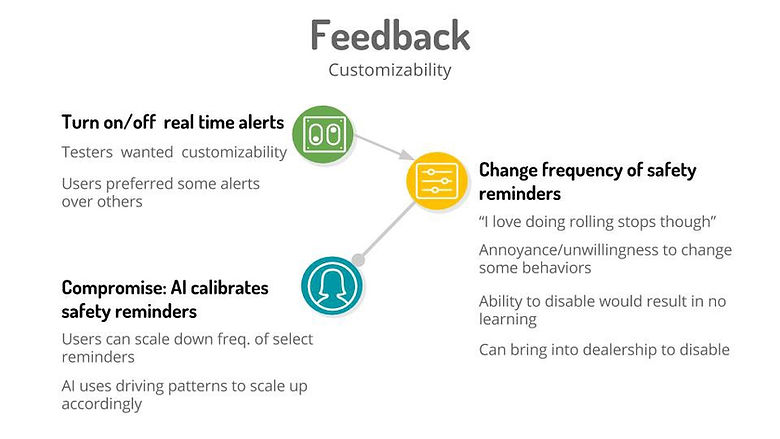
Based on our usability feedback, we decided to send out a survey to allow users to vote on preferred phrases and to allow for more customizability.. When revising the phrases, we opted for shorter phrases that communicated the message better. For dangerous or emergency situations, we chose to alert users through simple sounds like sharp beeps to trigger more rapid reactions. Some of the phrase changes we modified are depicted below:
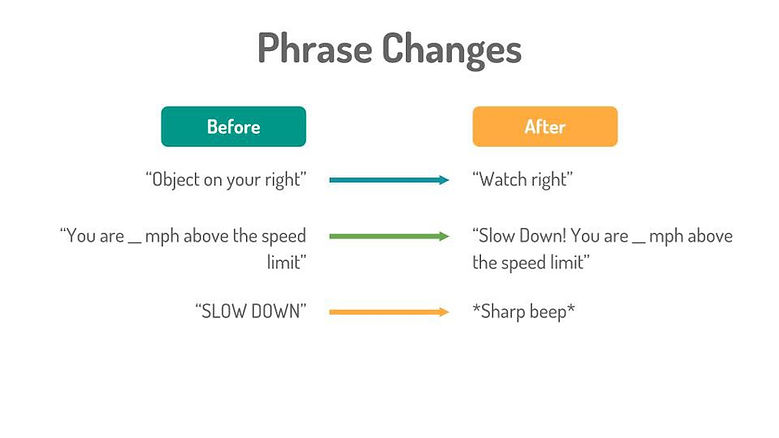
The features of MIA aims to use individuals' driving behaviors to customize effective audio feedback systems that assists drivers. MIA utilizes audio cues to reduce computational complexity to reinforce good driving habits and to keep the drivers' eyes on the road.
App Prototype
We needed to create an app to allow users to visualize data and customize their systems. Here is a link to all the screens consisting the prototype.
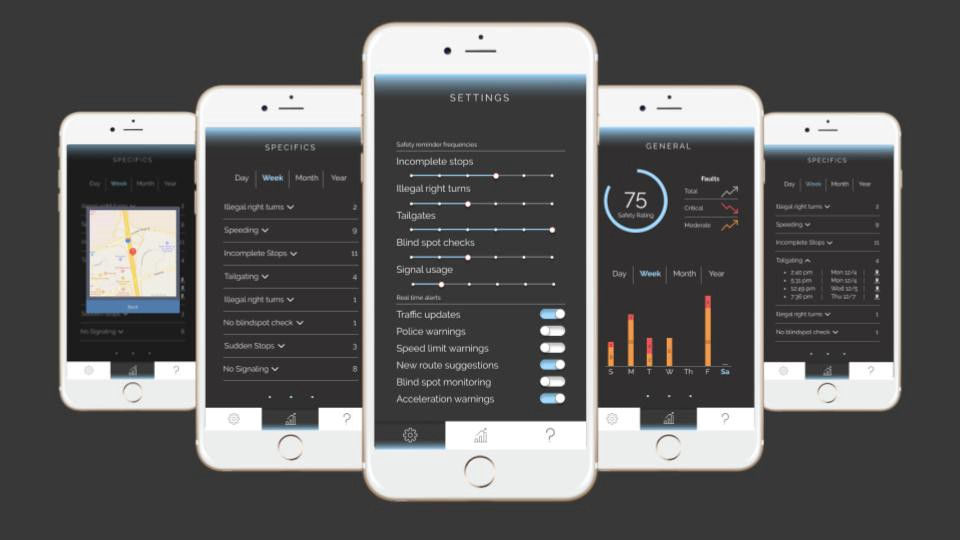